Article | 16 May 2019
AI in Health & Life Sciences & the Medicus L(ex) Machina
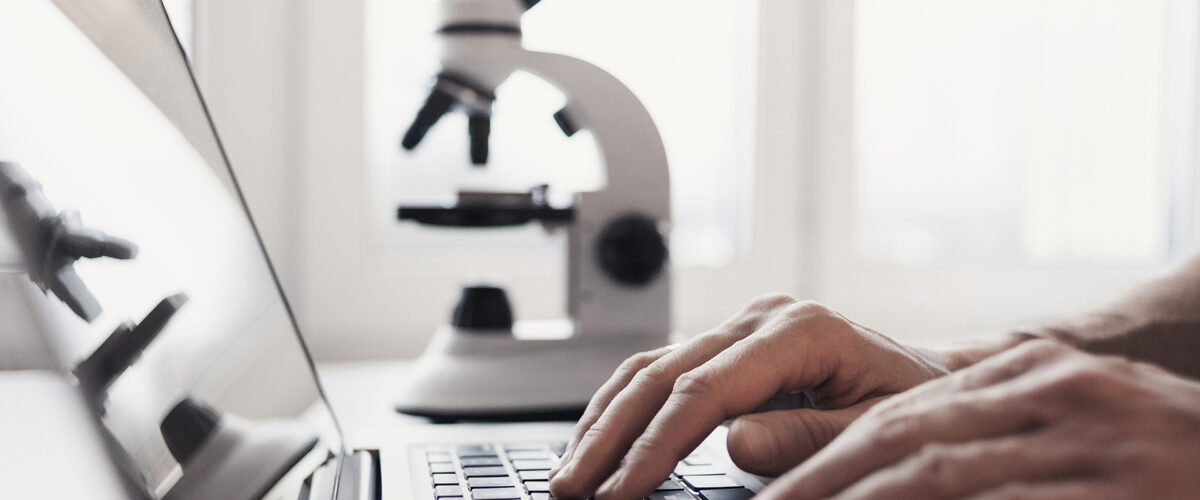
By Timo Minssen*
There is no doubt that Artificial Intelligence (AI) is at the centre of what is often referred to as the Fourth Industrial Revolution.(1) Although still in its infancy, we are already seeing enormous advances in a great variety of scientific and industrial areas. The impact of AI can be felt across industries and professional sectors, from military, transport and agriculture to transport, media, communication, entertainment and in the legal profession. Everything becomes smarter and interconnected. That surely also applies to health and life sciences.
Many companies and healthcare providers are investing heavily in developing AI services in health care. This has resulted in several applications that are already deployed in the healthcare industry, such as using chatbots to make urgent treatment decisions, AI-driven X-ray image analysis, smartphone apps that detect skin cancer with more accuracy than dermatologists, and the use of AI-driven monitoring to identify elderly patients at risk of falling or to predict the course of disease outbreaks. AI is also being applied to make drug discovery more effective and only recently, US authorities have approved the first smart pills – also referred to as ingestible electronic sensors – that send valuable information to medical practitioners, such as how the patient’s specific metabolism reacts with the drug and if the patient is actually taking the right dosage at the right time. This not only increases the safety of often elderly patients by monitoring their drug use; it may also help to optimise drug administration to control the use of e.g. antibiotics in the battle against antimicrobial resistance.
So far, AI is used as a tool. But imagine a world where patients are admitted to fully automated hospitals. Imagine a world where personalised drugs are first tested on our digital twins. Imagine a world where AI is teaching and improving itself in hospital and home-settings through deep machine learning and quantum computing. Imagine a world where AI is becoming creative in the medical sector, not only reaching a diagnosis, but actually executing the treatment decision in the emergency room and applying for patent protection for its inventions.
It is therefore clear that digital technologies, smart data, and AI will be both a beneficial foundational technology that creates marvellous opportunities, but also a disrupting force resulting in several crucial challenges for the health and life science sector. In the near future, these technologies will require us to find answers to more fundamental questions, such as: who is responsible when things go wrong? Who is the lord of the digital rings that bind them all? Who wields the profits and how? And who is the master and what is the place for humans and humanistic values in potential scenarios?
Lawyers might sometimes feel like “party crashers” when entering high-tech events and start-up scenes to ask critical questions. But without a doubt, digitalisation and AI raise fundamental societal, philosophical and legal questions. It appears therefore more important than ever to stress the significance of law and lawyers in this area. This also includes the realisation that competent legal advice is not only about identifying legal road-blocks to tell high-tech developers and clients what they cannot do under the current legal regime. Rather, we need skilled legal expertise to find creative solutions that may help to avoid problems further down the road, thereby helping to achieve sustainability, fairness, due process and competitiveness in the sector. And we need appropriate and internationally enforceable legal frameworks to promote the promises and regulate the perils of the amazing technological possibilities.
Probably the most important areas in which challenges and legal issues are frequently debated concern (1) the ethics of algorithmic decision-making, (2) the cybersecurity of AI systems, (3) the transparency and accountability of complex and opaque algorithmic decision-making, (4) the protection of privacy, particularly with regard to the General Data Protection Regulation (GDPR), (5) questions concerning intellectual property rights, competition law and data ownership, (6) inequality, bias and discrimination resulting from AI applications, (7) quality assurance for both data and AI-driven decision-making, (8) the usability and interoperability of data, (9) liability, and (10) trust.(2) In the following I will go through a few legal issues selected from this broad list.
Liability
Let’s start with the question of liability: what do we do when artificial intelligence in the health and life sciences makes mistakes and things go wrong? After all, there have been notable failures of AI, such as a USD 62 million project carried out by IBM for a US hospital that produced no tangible benefits and resulted in scepticism regarding AI in medicine. But things could get worse, as we have learned from the recent accidents involving self-driving cares. What if software bugs or cyber-attacks harm or kill patients, e.g. by falsifying image analysis(3) or exposing patients to far too high X-ray radiation?
So far, courts have been reluctant to apply traditional product liability theories to healthcare software and algorithms as such. Part of that reluctance has come from the fact that to date, healthcare software has been characterised primarily as a tool that helps healthcare providers reach decisions, with the final decision always resting in the hands of the provider.(4)
But AI-driven medicine contests that approach. How, can and should healthcare providers be fully responsible for treatments suggested or made by highly complex and opaque algorithms based on an automated analysis of enormous amounts of complex data that is not transparent?(5) How should they evaluate conclusions or implement decisions that they do not, or cannot, understand? Should we have incorporated liability regimes that have been written into the code of the algorithms? Should we have international funds and international frameworks to hold AI responsible and to pay out damages? These questions are not easy to address.
Moreover, there are additional problems at the decision-making level: do doctors, nurses, healthcare technicians etc. have a duty to question AI decisions? Medical doctors that have vowed to help patients in accordance with their best abilities and that have developed a sense of intuition based on years of experience will surely feel a strong obligation to intervene if an algorithm suggests an intervention that seems unhelpful, useless, expensive, or even dangerous.(6) But if they only implement those decisions they would have reached on their own, they will undermine much of the benefits that this new technology promises.(7)
In the future, things might become even more complicated when AI and machine learning begins to be self-learning and independently implements decisions in e.g. surgery. Who is responsible then? Courts have not tackled these issues yet, but they will have to in the near future and find legal answers to who will be held responsible if things go wrong. But before it comes to such failures, lawyers and the regulatory system should of course make sure that such disasters are prevented. And this brings me to the next area:
Regulatory challenges & cybersecurity
Medical authorities that approve new treatments are currently working intensively to figure out if – and if so, how – to interpret, apply or modify their existing guidelines and regulatory frameworks in the AI context.(8) Typical issues related to the question of how to categorize AI-driven technologies and apps as a “medical device”, which would trigger strict sector-specific obligations across complex value chains.(9) But cyber security is also a major issue here, which explains why many jurisdictions around the world have invested considerable resources and launched legal initiatives to increase the security of AI-driven systems.(10)
A related problem concerns so-called black box medical devices: how should authorities assess what they do not fully understand when they need to decide on the safety and efficacy of new AI-driven technologies using extremely complex data and secret algorithms in MA procedures?
This is a major concern for medical authorities, such as the European Medicines Agency (EMA) or the US Food and Drug Administration (FDA), which are intensively searching for experts who have not only experience in artificial intelligence and machine learning, but who also have an understanding of how this technology will evolve across complex value chains and life cycles. Moreover, several new regulations and legislative activities in the regulatory area, such as the EU’s new medical device regulation(11) or cybersecurity act,(12) will have to be monitored very carefully.
Privacy
In addition to safety and efficacy such experts will have to consider privacy and patient engagement. Privacy is important in at least two areas: collecting enormous amounts of healthcare data to develop algorithms, and sharing such data to monitor them.(13) In each case, patient-oriented data privacy is a concern.
Recently enacted European legislation (GDPR) places strict constraints on the type of patient and health data that can processed. To process health or biometric data at all, companies must either obtain explicit consent from a person or fall under various GDPR exceptions relating to medical treatment, clinical trials and public health. In addition much of the data needs to be anonymized and the questions are: 1) To what extent is that really possible and 2) How valuable will the data still be for medical purposes?
Irrespective of these challenges, many have applauded the GDPR for prioritising privacy and patient rights by establishing strict and enforceable requirements and the need for organisational compliance. Others highlight the importance of the wide availability of health data to promote healthcare innovation and would stress the negative impacts of heightened compliance thresholds on health innovation and AI (in particular for SMEs).
However, in light of recent examples of data misuse and the Facebook/Cambridge Analytica/Google scandals, it is clear to me that protecting privacy is extremely important. The GDPR also fosters a new environment where healthcare providers will compete on their capability to engage and seek the consent of those whose data they seek the most to get the best data quality. The GDPR aims to let patients control their data in a clear and transparent way. If new privacy laws drive competition in big-data research that still protects patient privacy and autonomy, that is progress. This will also help us promote public trust, which is absolutely needed if this technology is to be accepted by society.
Transparency & Trust
Promoting public trust will be absolutely crucial to achieve the acceptance of AI technology by the regulatory system and the market. Among other things, this requires the protection of privacy, but also a reasonable degree of transparency with regard to the data that is used by the algorithm and the logic that applies to it. It will be absolutely vital to achieve an appropriate balance between these sometimes conflicting values.
This has also been recognised by policy makers, political institutions and authorities on both sides of the Atlantic, such as the EU Commission and medical authorities that have started initiatives to increase transparency and portability of data, and to develop data infrastructures that will improve our possibilities to interconnect and share data. Furthermore, it will be important to consider new processes that would allow AI users to question and sometimes challenge the decisions made by AI.(14)
Much emphasis has also recently been placed on the need to improve data quality, to make health and clinical data more transparent, and to make sure that such data is findable, accessible, interoperable, and reusable. This is incredibly important as “Big Data” and “Smart Data” are the fuel of artificial intelligence and digitalisation. This also explains why a recent Economist series described “Big Data” as the new oil.
This brings us to the next issue:
Protection and governance of intellectual property
Considering that “Big Data is the new oil” or even “better than oil”,(15) it is easy to imagine that many wars will be fought over it. In business these wars will involve – in addition to rules on privacy protection – legal forms of protection, such as a great variety of overlapping and interacting intellectual property rights and trade secrets. These rights do not only act as incentives; they also often have exclusionary effects and might stand in the way of the free availability of data.
In addition, we will have to ask: what type of computer and AI-implemented inventions should be considered patentable?(16) Who will be allowed to seek protection for their inventions? Should AI have a legal identity and be allowed to seek IP protection for AI-created inventions? Does it need incentives? What will be the role of humans in innovation and who will be able to reap the profits of protected innovation? There are many questions for which legal research and legislative decisions are indeed needed. And this is also true of the next, very much related topic:
Data sharing and competition law
Many companies, including big pharmaceutical companies, realise that sometimes data needs to be shared and collaboration is necessary to develop the next generation of treatments and to compete in a rapidly developing market. Yet, they might often want to control – and sometimes with good reason – which data is shared, under what conditions it is shared and with whom it is shared.
It is then our obligation as legal experts to think about how we can create legal frameworks that enhance and incentivize the sharing of such high-quality data. And where companies merge, act unfairly or collude to completely control a market where competition and access is vital for the benefit of society, we need to find better ways to intervene with refined tools of competition law (in the EU under Articles 101 & 102 of the TFEU). A more dynamic competition also implies that competition law and competition lawyers will have to become more forward looking and will e.g. have to understand big data and AI concepts better. This is highlighted e.g. in a recent March 2019 report from the EU Commission.(17)
Where the denial of access to data is concerned, this includes the realisation that not all data is created equal and that the value of data depends on several factors, including its use and whether the data is unique. For example, because machine learning is a process of dynamic experimentation, varied data that offers a multiplicity of signals tends to be more useful.(18) Turning to a merger and acquisitions context, it is e.g. theoretically possible that certain combinations in which consumer data is a key asset could lead to market power, but this outcome seems less likely unless it is shown that the data could not be replicated or has no substitutes.(19)
Societal challenges, ethics, justice & fairness
Let’s turn to one of the most important issues by far: ethics, justice and fairness. Many legal issues are dwarfed by the broader societal and ethical issues posed by AI in the health and life sciences. If AI can do almost everything better, we also have to ask what will happen to the human work force, including doctors and lawyers. History has shown that society has adapted to industrial evolution by replacing old jobs with new responsibilities. But the emergence of AI takes this to a new level. At some point we need to make serious political decisions on how to distribute the wealth created by AI and on how to take care of those who have not found a new task in society. Likewise, regulatory frameworks must evolve to address these issues and the legal and philosophical concept of human rights, “human dignity and equal value” is of the utmost importance here.
Another issue concerns bias and discrimination. In the wider future AI and big data will surely glocalize health care and bring it to areas that are remote, thereby helping us to address crucial societal problems. Yet, any algorithm will only be as good as the data that it is trained with, and – before it becomes autonomous – AI will depend on world-class experts to apply the technology. The problem is that training algorithms to recommend treatments that work in world-class hospitals may often mean that those algorithms don’t recommend appropriate and cost-effective treatments in less elite settings. Hence there is a great risk for bias and discrimination. Social, medical and legal research should therefore explore the risk of such biases so that algorithms that are applied in low-resource contexts do not create problems in medical care for those who need it most.(20) These and other data ethics questions are at the top of the agenda for many national and international policies. It can be expected that this will result in ethics standards and ethics seals, which might become a competitive factor in the emerging data economy. At the same time, it is evident that while ethics can certainly provide blueprints and guidelines for a socially responsible and beneficial use of digital technology, it cannot always implement them. In particular, with regard to responsible solutions that require major investments and regulatory control, it will be crucial that ethical guidelines are accompanied by enforceable laws.
Conclusions
AI is not only disrupting industrial value chains; it is also transforming society. Researchers, but also other important stakeholders such as law firms, industry, politicians, patient organisations etc. have an absolutely crucial role to play in this trajectory.
Many of the problems can only be addressed if we break disciplinary silos of research and business and we need to have the right incentives and frameworks in place to succeed in the process. Interdisciplinary research, wider stakeholder outreach and collaboration across sectors and industries is absolutely crucial if we want to prepare the next generation, including lawyers, for the future and help them find their place and their professions. They will face extremely complex debates and challenges and it is our obligation to prepare them. This should not only involve the teaching of computational thinking and so-called legal tech. The humanities are at least as important since they define us. This means that we should not only digitalise the law; we must also legalize digitalisation. Sometimes the law and ethical guidelines must evolve to set up barriers; sometimes the law must be adapted so we can enable and support these developments. And we need internationally enforceable frameworks for this.
Moreover, we better make sure that we implant our humanistic principles and values, as well as the rule of (fundamental) laws into the evolving AI landscape. Before AI takes over, this also means that we need to create emergency breaks and emergency exits. And, finally, we should be very careful not to become too dependent on AI in the future – let’s keep our old skills sharp. Because after AI is gone, how should we carry on?
———————————————————————-
*Professor of Law, Founding Director of the Centre for Advanced Studies in Biomedical Innovation Law (CeBIL) at the University of Copenhagen; Permanent Visiting Professor, Lund University. This contribution is a modified version of a talk prepared for the annual celebration of the University of Copenhagen on 19 November 2019 (Årsfest festforelæsning: Welcome to the Machine! – Artificial Intelligence, Health Innovation & the Law). CeBIL’s research is supported by a Novo Nordisk Foundation grant for a scientifically independent Collaborative Research Programme (grant number NNF17SA027784).
(1) Some might prefer to call it the First Digital Revolution.
(2) These core issues are often discussed in combination with calls to modernise legislation, to improve public communication on AI matters, to enhance the education and competences of our Scandinavian workforce with regard to computational thinking, to adjust legal and regulatory procedures, as well as to reorganise crucial sectors, such as health care.
(3) Mirsky et al., CT-GAN: Malicious Tampering of 3D Medical Imagery using Deep Learning, available at: https://arxiv.org/pdf/1901.03597.pdf (accessed 10 May 2019).
(4) Nicholson Price, Artificial Intelligence in Health Care: Applications and Legal Implications, available at: https://repository.law.umich.edu/cgi/viewcontent.cgi?article=2932&contex… (accessed 10 April 2019).
(5) Id.
(6) Id.
(7) Id.
(8) Minssen, Timo, An EU perspective on the Future of AI in Personalized Healthcare: What are the most pressing legal challenges and where can they be found? International Congress on Personalized Health Care – Montreal 23/09/2018 → 26/11/2018.
(9) Minssen Timo, Precision Medicine, Artificial Intelligence and the Law, Talk at CeBIL’s Annual Symposium 2018, Cambridge, see: https://www.publicpolicy.cam.ac.uk/events/pp-annual-symposium (accessed 10 May 2019).
(10) Gerke, S, Minssen, T, Yu, H & Cohen, G 2019, A Smart Pill to Swallow: Ingestible Electronic Sensors, Cutting Edge Medicine, and Their Legal and Ethical Issues (commissioned by Nature Electronics & submitted and under review).
(11) Regulation (EU) 2017/745 of the European Parliament and of the Council of 5 April 2017 on medical devices, amending Directive 2001/83/EC, Regulation (EC) No 178/2002 and Regulation (EC) No 1223/2009 and repealing Council Directives 90/385/EEC and 93/42/EEC [2017] OJ L117/1.
(12) Proposal for a regulation of the European Parliament and of the Council on ENISA, the “EU Cybersecurity Agency”, and repealing Regulation (EU) 526/2013, and on Information and Communication Technology cybersecurity certification (“Cybersecurity Act”) (COM(2017)0477 – C8-0310/2017 – 2017/0225(COD)), 12 March 2019.
(13) Nicholson Price; Kaminski, Margot; Minssen, Timo & Kayte Spector-Bagdady, Shadow health records meet new data privacy laws, in: SCIENCE 2019; Vol. 363, No. 6426. pp. 448-450.
(14) Wachter, Sandra and Mittelstadt, Brent, A Right to Reasonable Inferences: Re-Thinking Data Protection Law in the Age of Big Data and AI (23 April 2019). Columbia Business Law Review, 2019(1). Available at SSRN: https://ssrn.com/abstract=3248829
(15) Some might argue that big data is actually better than oil, since “data trumps the value of oil in so many ways: it is in effect infinitely durable and reusable; can be replicated indefinitely and instantly moved around the world at a very low cost; becomes more useful the more it is used and once processed, data often reveals further applications. As well, data variety is endless: it can represent words, pictures, sounds, ideas, facts, measurements, statistics or anything else that can be processed by computers into strings of 1s and 0s that make up digital information”, see: Ieva Giedrimaite, The end of code – long live data, available at: http://ipkitten.blogspot.com/2019/04/the-end-of-code-long-live-data.html (accessed 10 May 2019).
(16) See e.g. Chapter 3.3.1 of the new EPO Guidelines on Artificial intelligence and machine learning (November 2018, available at: https://www.epo.org/law-practice/legal-texts/html/guidelines2018/e/g_ii_… (accessed 10 May 2019). Minssen, T & Pierce, J 2018, Big Data and Intellectual Property Rights in the Health and Life Sciences, in IG Cohen, H Fernandez Lynch, E Vayena & U Gasser (eds), Big Data, Health Law, and Bioethics. Cambridge University Press, Cambridge, pp. 311-323, 2016.
(17) Jacques Crémer, Yves-Alexandre de Montjoye, Heike Schweitzer, Competition Policy for the digital era, Final Report for the European Commission (2019), available at: http://ec.europa.eu/competition/publications/reports/kd0419345enn.pdf. See also EU Commission Report, April 2019, Competition Enforcement in the Pharmaceutical Sector (2009-2017), available at: http://ec.europa.eu/competition/publications/reports/kd0718081enn.pdf.
(18) See Elena Kamenir, Themes and Takeaways from the FTC Hearings on the Intersection of Big Data, Privacy, and Competition, available at https://www.competitionpolicyinternational.com/wp-content/uploads/2018/1… (accessed 10 January 2019).
(19) Id.
(20) Price, William Nicholson, Medical AI and Contextual Bias (March 8, 2019). Harvard Journal of Law & Technology, Forthcoming. Available at SSRN: https://ssrn.com/abstract=3347890 .
Contact:
Practice areas: