Article | 20 Nov 2024
Liability, Opportunity and Risk: THE use of Generative AI in the Finance Industry
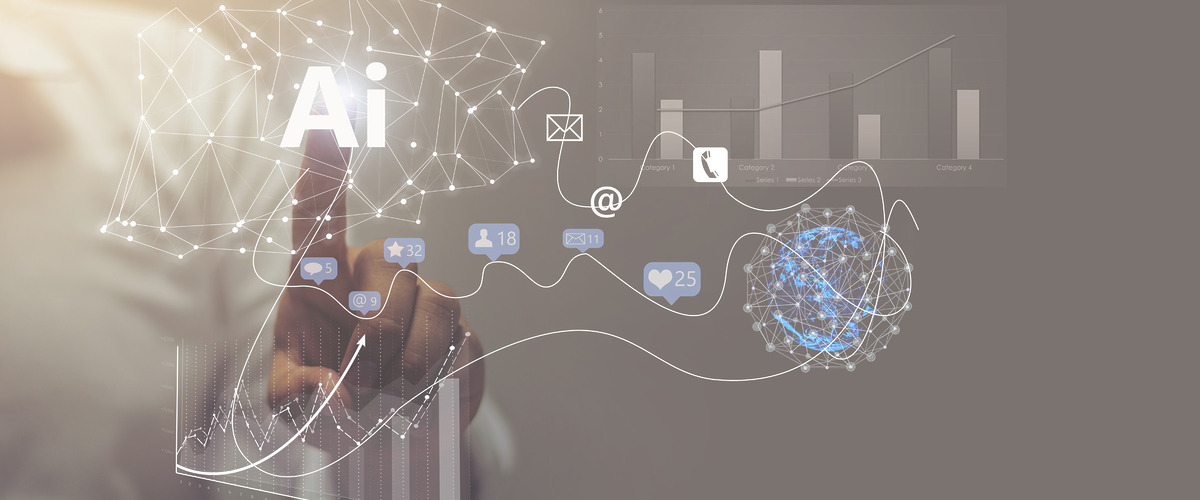
The finance industry is no stranger to new technological breakthroughs, which typically come at such speed and with such ferocity that life is never quite the same again thereafter. The finance industry is yet again on the verge of another radically transformative era, with the evolution of generative artificial intelligence (“AI”). In its current iteration, AI already offers tools capable of everything from automating routine tasks to enhancing decision-making processes, and creating new strategies. These opportunities are indeed tempting, and businesses who are late to the AI game may be left behind competitors. However, implementing generative AI also comes with significant risks and liability concerns that must be carefully navigated.
Opportunities Presented by Generative AI
Automation of Financial Reports and Analysis: Generative AI can automate the creation of financial reports, executive summaries, and personalised investment insights. This not only increases efficiency but also allows financial analysts to focus on more strategic tasks.
Personalized Financial Services: By leveraging generative AI, financial institutions can offer highly personalised services to their clients. AI can generate customised investment strategies, risk assessments, and even financial advice tailored to the individual needs of customers.
Fraud Detection and Prevention: Generative AI can be trained to identify patterns indicative of fraudulent activity. By analysing transactions in real-time, AI systems can flag anomalies and prevent potential fraudulent activity before it even occurs.
Algorithmic Trading: AI algorithms can generate predictive models that drive algorithmic trading strategies. These models can process vast amounts of market data to identify trading opportunities that may be invisible to human analysts.
Risks and Liability Concerns
Model Risk and Transparency: The complexity of generative AI models can lead to a lack of transparency, making it difficult to understand how decisions are made. This ‘black box’ issue can pose significant model risks, especially if the AI generates erroneous or biased outputs. The lack of transparency, as well as the tendency for Generative AI to ‘hallucinate’, poses difficulties for financial institutions and their ability to demonstrate security and accountability, and thereby ensure regulatory compliance.
Regulatory Compliance: As financial institutions adopt generative AI, they must ensure that their use of the technology complies with existing regulations. This includes everything from the strict requirements in DORA and NIS2 regarding cybersecurity as well as the EU AI Act, but also more mundane legal requirements on everything from job application discrimination, ensuring the accuracy of generated reports, use of third-party intellectual property rights (either in the prompting phase or as part of the generated result), etc.
Data Privacy and Confidentiality: Generative AI requires access to large datasets, which may include sensitive personal and financial information. Ensuring the privacy and security of this data is paramount in order to prevent breaches that could lead to significant liability issues.
In relation to prompting and sharing information as a financial institution or from third parties (e.g., customers, trusted partners, or suppliers), many AI tools have features and/or legal terms that mean that the provider of the tool has access to and uses such information to, for example, further develop and improve the tool or other services. This may mean that the instructions become available both to the provider of the tool and other unauthorised third parties, despite, and in breach of strict confidentiality undertakings by the financial institution.
Navigating the Balance
To harness the benefits of generative AI while simultaneously mitigating the associated risks, financial institutions must adopt a balanced approach.
Governance Framework: Establishing strong governance frameworks will help manage the risks associated with generative AI. This includes setting clear policies for model development, validation, and deployment but also for the purchase of third-party AI tools and their use within the organisation – including when and how employees may use confidential information and personal data when prompting an AI tool. A multi-level and cross-functional approach is imperative to ensure that all actors are aware of their roles and responsibilities, and to ensure compliance with applicable law (e.g., the EU AI Act), and other legal obligations undertaken by the financial institutions (e.g., as part of a collaboration agreement with a supplier).
Review of Legal Terms: Generative AI tools are software programs under legal terms often supplied by the provider of the tool. To understand the risks with a specific tool, and to mitigate such risks, all financial institutions need to implement routines for the review of applicable terms and conditions of all third-party AI tools.
Conclusion
The use of generative AI in the finance industry offers a compelling blend of opportunities and challenges. Financial institutions that successfully integrate this technology can reap significant rewards in terms of efficiency, customer satisfaction, and competitive advantage. However, they must also be vigilant in addressing the risks and regulatory considerations that come with it. By implementing robust risk management practices in the form of inter alia governance frameworks and routines to review legal terms regulating use of generative AI by the organisation, the finance industry can navigate the complexities of generative AI and savour its opportunities with minimal risks.